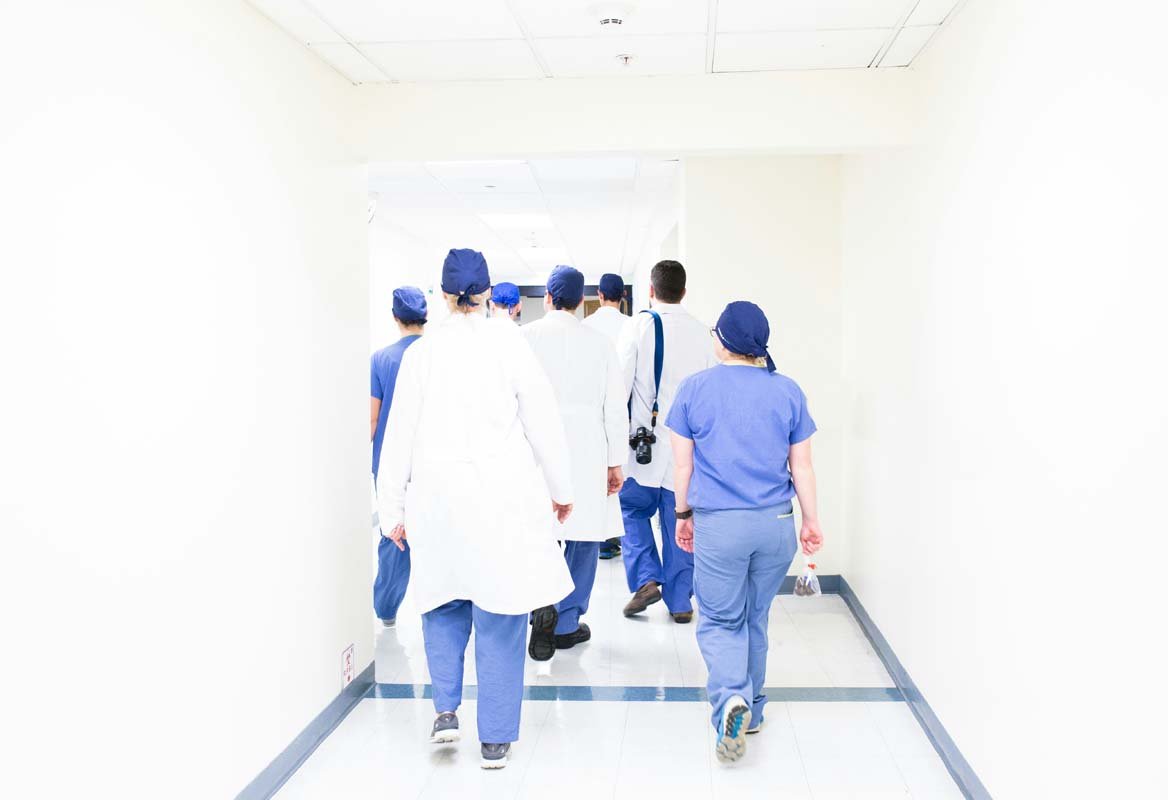
Project Activities
Generic drugs play a crucial role in providing affordable healthcare solutions. However, the development process can be lengthy and costly due to stringent regulatory requirements and complex formulation challenges. This project seeks to harness AI and machine learning to streamline the development of generic drugs, accelerating the process and reducing costs.
Objectives:
- Develop AI models to optimize drug formulation processes.
- Use machine learning to predict and mitigate regulatory hurdles.
- Enhance the efficiency of bioequivalence studies through AI.
- Integrate AI tools into existing drug development workflows.
- Conduct pilot studies to validate the effectiveness of the AI-enhanced processes.
- Share findings and best practices with the pharmaceutical industry.
Methodology:
- Data Collection: Gather comprehensive datasets from existing generic drug development projects, including formulation, bioequivalence, and regulatory data.
- Model Development: Develop AI and machine learning models to optimize formulation processes and predict regulatory challenges.
- Integration: Work with pharmaceutical companies to integrate AI tools into their existing workflows.
- Pilot Testing: Conduct controlled trials to test and refine the AI-enhanced processes.
- Evaluation: Analyze pilot study data to assess improvements in development time, cost, and regulatory success rates.
- Dissemination: Share results through publications, workshops, and conferences, providing training materials for broader implementation.
Beneficiaries:
- Patients: Improved access to affordable medications.
- Pharmaceutical Companies: Reduced development costs and time to market.
- Regulatory Agencies: Streamlined approval processes with more predictable outcomes.
- Healthcare Providers: Greater availability of cost-effective treatment options.
- Insurance Companies: Lower drug costs and increased savings.
- Policy Makers: Data-driven insights to support healthcare policy and regulation.
Project Milestones:
- Month 1-2: Planning and Setup
- Establish project team and roles.
- Develop detailed project plan and timeline.
- Secure partnerships with pharmaceutical companies.
- Month 3-5: Data Collection and Preparation
- Aggregate datasets from existing generic drug development projects.
- Conduct initial data cleaning and preprocessing.
- Month 6-8: Model Development
- Develop AI models for optimizing drug formulation processes.
- Perform iterative testing and refinement of models.
- Month 9-11: Integration with Development Workflows
- Collaborate with pharmaceutical companies for system integration.
- Ensure interoperability and user-friendly interfaces.
- Month 12-14: Pilot Testing
- Conduct pilot studies to test and refine AI-enhanced processes.
- Collect data and feedback for further improvements.
- Month 15-17: Evaluation
- Analyze pilot study results to assess impact on development time, cost, and regulatory success rates.
- Make necessary adjustments based on findings.
- Month 18-20: Final Implementation
- Finalize AI models and integration processes.
- Deploy the tools in broader pharmaceutical settings.
- Month 21-24: Dissemination and Training
- Share findings through publications, workshops, and conferences.
- Provide training materials and sessions for stakeholders.
This project aims to transform the generic drug development process by leveraging AI and machine learning, ultimately making medications more accessible and affordable for patients worldwide.
project beneficiaries
Patients
Pharmaceutical Companies
Regulatory Agencies
Healthcare Providers
Insurance Companies
Policy Makers
brief description
This project aims to leverage AI and machine learning to streamline the development of generic drugs, reducing costs and time to market. By optimizing formulation processes and predicting regulatory hurdles, we seek to enhance drug accessibility and affordability for patients.